AI has had a huge impact on many industries in recent years, changing the way we work with and process data. One of the biggest advances in Artificial Intelligence AI Algorithms in edge computing. Edge computing refers to the practice of processing and analyzing data at the edge of the network, closer to where it is generated.
This approach minimizes latency and reduces the need for transferring large amounts of data to the cloud or centralized servers. In this article, I will delve into the future of intelligent edge computing, focusing specifically on the role of AI algorithms in this evolving field.
Table of Contents
Understanding AI algorithms in edge computing
Before we explore the potential of AI algorithms in edge computing, it is crucial to understand what these algorithms are and how they work. AI algorithms are mathematical formulas or sets of rules designed to enable machines to learn from data, make decisions, and perform tasks without explicit programming.
These algorithms can be trained using large datasets, allowing them to recognize patterns, make predictions, and optimize processes.
In the context of edge computing, AI algorithms play a vital role in enabling devices at the edge to perform intelligent tasks. For example, AI algorithms can be used to analyze sensor data in real-time, identify anomalies, and trigger appropriate actions. These algorithms can also be deployed to optimize resource allocation, enhance security measures, and improve overall efficiency.
Benefits of using AI algorithms in edge computing
The use of AI algorithms in edge computing offers numerous benefits across various industries. Firstly, it reduces latency by processing data locally, ensuring faster response times and enabling real-time decision-making. This is particularly crucial in applications that require immediate actions, such as autonomous vehicles, industrial automation, and healthcare monitoring systems.
Secondly, AI algorithms in edge computing minimize the reliance on cloud resources, leading to cost savings and improved scalability. By performing data processing and analysis at the edge, organizations can significantly reduce bandwidth requirements and avoid potential network congestion issues.
This distributed approach also enhances data privacy and security, as sensitive information can be processed locally without being exposed to external networks.
Lastly, AI algorithms in edge computing enable offline capabilities, allowing devices to continue functioning even when they are disconnected from the cloud or experiencing network disruptions. This is particularly valuable in remote and harsh environments where connectivity may be intermittent or unreliable.
Current applications of AI algorithms in edge computing
The implementation of AI algorithms in edge computing is already seeing practical applications across various industries. In the healthcare sector, AI algorithms are being used to analyze patient data collected by wearable devices, enabling early detection of health issues and timely interventions. This real-time analysis at the edge can potentially save lives by providing immediate alerts to medical professionals.
In the manufacturing industry, AI algorithms in edge computing are optimizing production processes by monitoring equipment health, predicting failures, and scheduling maintenance activities. This proactive approach reduces downtime, increases productivity, and improves overall operational efficiency.
Furthermore, in the transportation sector, AI algorithms are being leveraged in autonomous vehicles to process sensor data and make split-second decisions. This real-time analysis at the edge ensures safe and reliable autonomous navigation, enhancing road safety and revolutionizing the future of transportation.
Challenges and limitations of AI algorithms in Edge Computing
While the potential of AI algorithms in edge computing is promising, there are several challenges and limitations that need to be addressed. One of the main challenges is the limited computational power and storage capacity of edge devices.
AI algorithms often require significant computational resources, which may not be available on resource-constrained edge devices. This limitation calls for the development of lightweight AI algorithms that can efficiently run on edge devices without compromising accuracy and performance.
Another challenge is the need for effective data management strategies. Edge devices generate vast amounts of data, and it is crucial to determine which data should be processed locally and which data should be sent to the cloud for further analysis. This decision-making process requires intelligent algorithms that can prioritize data based on relevance, importance, and available resources.
Furthermore, ensuring the security and privacy of data processed at the edge is a significant concern. Edge devices are more susceptible to physical tampering and cyber-attacks, making it essential to implement robust security measures to protect sensitive information. AI algorithms need to be designed to detect and mitigate potential security threats, ensuring the integrity and confidentiality of data.
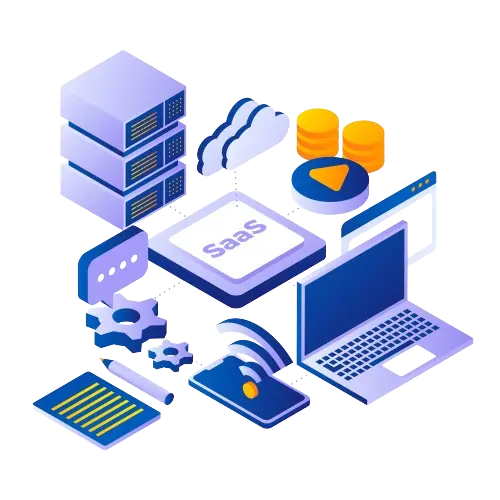
The future of intelligent Data illustrations by Storyset
Looking ahead, the future of intelligent edge computing holds immense potential. As technology continues to advance, we can expect AI algorithms to become more sophisticated and capable of performing complex tasks at the edge. This advancement will enable edge devices to make more intelligent decisions, adapt to changing environments, and learn from real-time data.
One area where AI algorithms in edge computing will have a significant impact is in the Internet of Things (IoT) ecosystem. With the proliferation of IoT devices, the amount of data generated at the edge is growing exponentially.
AI algorithms will play a crucial role in processing and analyzing this data in real-time, enabling organizations to derive valuable insights and make informed decisions.
Moreover, as 5G networks become more widespread, edge computing will become even more prevalent. The low latency and high bandwidth of 5G will further enhance the capabilities of AI algorithms at the edge, enabling real-time applications that were previously not feasible.
This convergence of 5G and AI algorithms will unlock new possibilities in areas such as augmented reality, smart cities, and autonomous systems.
Implementing AI algorithms in edge computing: Best practices
To fully leverage the potential of AI algorithms in edge computing, organizations should follow certain best practices. Firstly, it is crucial to carefully select and design AI algorithms that are suitable for edge deployment. Lightweight algorithms that can run efficiently on resource-constrained devices should be prioritized.
Additionally, algorithms should be optimized for low power consumption to prolong the battery life of edge devices.
Secondly, organizations should invest in robust edge infrastructure that can support the computational requirements of AI algorithms. Edge servers, gateways, and edge accelerators should be deployed strategically to ensure optimal performance and scalability. This infrastructure should also incorporate security measures to protect against potential threats.
Lastly, organizations should adopt a hybrid approach that combines edge computing with cloud resources. While AI algorithms at the edge offer numerous advantages, there are certain tasks that are better suited for the cloud. By leveraging both edge and cloud resources, organizations can achieve a balance between real-time processing and the scalability of cloud computing.
Industries that can benefit from AI algorithms in Edge-Computing
Numerous industries can benefit from the integration of AI algorithms in edge computing. One such industry is healthcare, where real-time analysis of patient data at the edge can enable remote monitoring, early detection of diseases, and personalized treatment plans. This has the potential to revolutionize healthcare delivery, especially in rural or underserved areas.
The manufacturing industry can also leverage AI algorithms in edge computing to optimize production processes, reduce downtime, and improve quality control. Real-time analysis of sensor data can identify potential issues before they escalate, ensuring uninterrupted operations and minimizing losses.
Additionally, the transportation industry can benefit greatly from AI algorithms at the edge. Autonomous vehicles can process sensor data in real-time, enabling safe and efficient navigation. This technology has the potential to transform the way we travel, reducing accidents and congestion while increasing accessibility.
The role of AI algorithms in enhancing Edge-Computing performance
AI algorithms play a crucial role in enhancing the performance of edge computing systems. By enabling intelligent decision-making at the edge, AI algorithms reduce the need for data transfer to the cloud or centralized servers. This minimizes latency, ensures real-time responsiveness, and conserves network bandwidth.
Furthermore, AI algorithms can optimize resource allocation in edge computing systems. By analyzing data and predicting future demand, these algorithms can allocate computational resources efficiently, ensuring optimal performance and scalability. This proactive approach minimizes wastage and maximizes the utilization of resources.
Moreover, AI algorithms can enhance the security of edge computing systems. By continuously monitoring data and detecting anomalies, these algorithms can identify potential threats and trigger appropriate actions. This proactive security approach ensures the integrity and confidentiality of data processed at the edge.
Conclusion: The potential impact of AI algorithms on the future of Edge-Computing
In conclusion, the future of intelligent edge computing looks promising, with AI algorithms playing a crucial role in enabling real-time decision-making, reducing latency, and enhancing overall performance.
The integration of AI algorithms in edge computing offers numerous benefits across various industries, including healthcare, manufacturing, and transportation.
However, there are also challenges and limitations that need to be addressed, such as limited computational power, data management, and security concerns.
By following best practices and leveraging a hybrid approach that combines edge and cloud resources, organizations can fully capitalize on the potential of AI algorithms in edge computing. As technology continues to advance and 5G networks become more prevalent, the capabilities of AI algorithms at the edge will further expand, unlocking new possibilities and transforming industries.
The future of intelligent edge computing is bright, and as AI algorithms continue to evolve, we can expect to see even more innovative applications and advancements in this exciting field. It is an exciting time to be at the forefront of this technological revolution, and the potential impact on industries and society as a whole is immense.
Embracing AI algorithms in edge computing will undoubtedly shape the future of technology and pave the way for a more intelligent and connected world.
CTA: Discover how AI algorithms in edge computing can revolutionize your industry. Contact us today to explore the possibilities and stay ahead of the competition.