Data analysis is an essential part of the business world today. Companies in different sectors use data analysis to make informed decisions, gain insightful information, and fuel growth.
Through the analysis of vast amounts of data, companies can discover trends, patterns, and correlations that can be used to streamline their operations, enhance customer experiences, and fuel innovation.
Conventional data analysis techniques are often inadequate in delivering complete insights. This is where multimodal AI steps in.
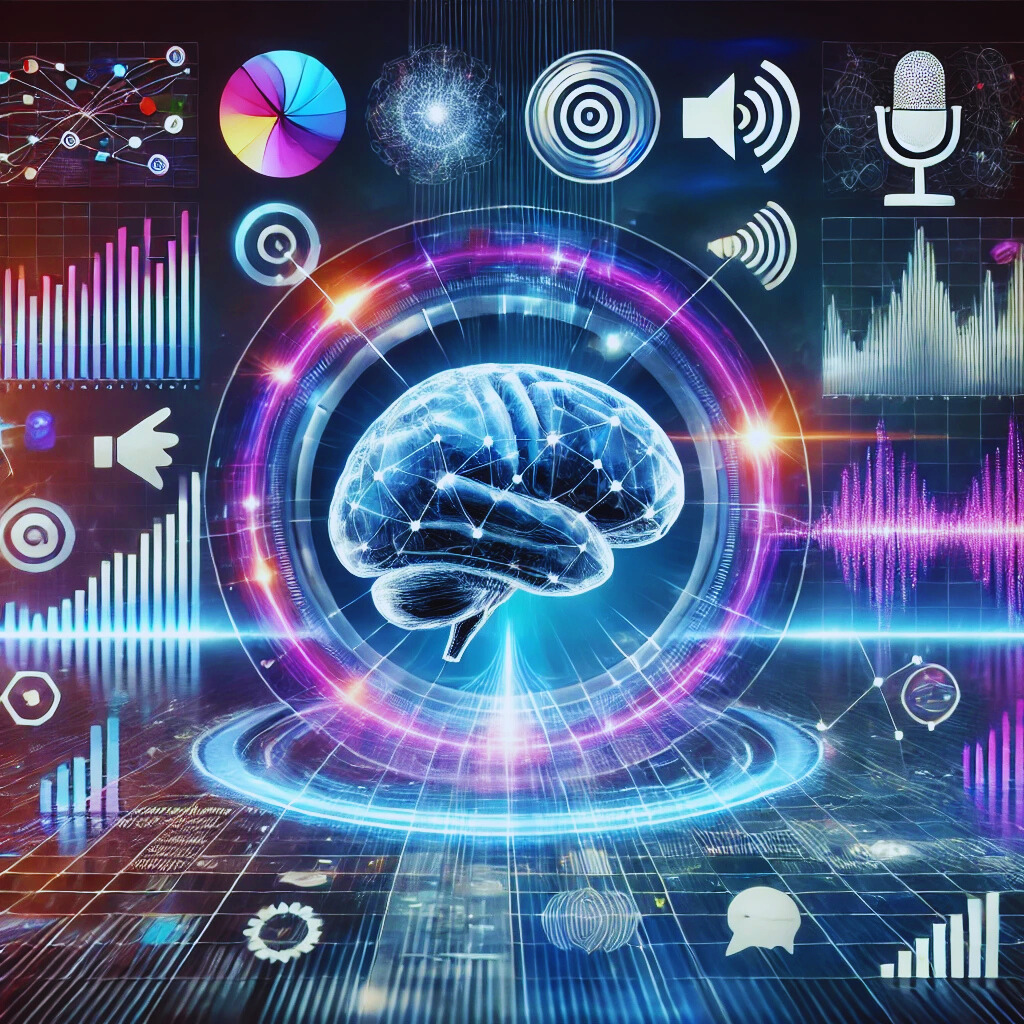
Table of Contents
Understanding visual analytics and its benefits
Visual analytics is the process of interpreting and examining data in visual form, like graphs, charts, and maps.
It makes it easy for companies to spot trends, outliers, and patterns in the data that might not be immediately obvious in unprocessed data. Visual analytics helps decision-makers to rapidly comprehend intricate information and make informed decisions.
Through visual analytics, companies can discover hidden insights, spot where they can improve, and present data effectively to stakeholders.
One of the most important advantages of visual analytics is that it can support exploratory data analysis.
Rather than using pre-defined queries or hypotheses, visual analytics enables users to interact with data in real-time, allowing them to discover new relationships and patterns that they might not have anticipated.
This interactivity and flexibility enable businesses to look at data from various perspectives and better understand their operations, customers, and markets.
Investigating speech analytics and its uses
Speech analytics is the analysis of spoken words to yield useful information and insights. It entails the transcribing of audio recordings of conversations into text and then subjecting it to natural language processing (NLP) to obtain meaning and sentiment.
Speech analytics allows companies to study customer interactions, for example, phone calls or recorded meetings, to understand customer preferences, behaviors, and moods.
One of the key uses of speech analytics is customer service. By examining interactions with customers, companies can see frequent pain points, enhance the performance of their agents, and enhance the overall customer experience.
Speech analytics also finds use in monitoring compliance, fraud detection, and the discovery of emerging issues and trends.
The strength of multimodal AI in data analysis
Multimodal AI blends visual and speech analytics to give a better, more integrated view of data analysis.
With the blending of these two modalities, companies are able to discover insights that can’t necessarily be gleaned by looking at the data individually.
Multimodal AI utilizes sophisticated machine learning algorithms to process and analyze visual and speech data and allow companies to draw relevant insights from any variety of sources.
Combining visual analytics and speech analytics for holistic insights
When speech and visual analytics are combined, companies can better understand their data and derive rich insights. By comparing visual and speech data, companies can discover patterns and correlations that are not visible when analyzing them in isolation.
For instance, by examining customer interactions using speech analytics and correlating them with visual data, companies can derive insights into customer behavior, emotions, and preferences.
Case studies highlighting the efficacy of multimodal AI for data analysis
There have been a number of case studies to highlight the efficacy of multimodal AI for data analysis. For instance, in the healthcare sector, multimodal AI has been employed to process medical images and patient records concurrently.
Through a combination of visual analytics and speech analytics, doctors can spot trends and correlations that can assist with diagnosis, treatment planning, and monitoring of patients.
In the retail sector, multimodal AI has been applied to understand customer behaviors and tastes by integrating visual information from security cameras with speech information from customer interactions.
This has enabled retailers to understand customer tastes in depth, optimize store layouts, and tailor marketing campaigns.
Tools and technologies for applying multimodal AI in data analysis
Multimodal AI deployment in data analysis involves the use of advanced tools and technologies. Some of the tools and technologies used include machine learning algorithms, natural language processing methods, deep learning libraries, and data visualization software.
There are various software platforms and libraries, including TensorFlow, PyTorch, and Tableau, that offer the tools and libraries needed to deploy multimodal AI in data analysis.
Best practices for combining visual and speech analytics
To properly utilize visual and speech analytics in combination, companies should keep the following best practices in mind:
Define clear objectives: Clearly state the objectives and goals of the data analysis project so that the combination of visual and speech analytics is in line with the intended outcomes.
- Ensure data quality: Make sure that the visual and speech data used for analysis are of high quality and representative of the target population or sample.
- Use optimal algorithms and methods: Choose the most suitable machine learning algorithms and natural language processing methods to analyze and process the visual and speech data efficiently.
- Validate and interpret results: Validate the results of the integrated analysis and interpret them in the light of the business goals.
- Challenges and limitations of integrating visual and speech analytics
Although combining visual and speech analytics has the potential to yield rich insights, there are a number of challenges and limitations to be considered.
One is the multimodal data processing and analysis complexity that demands specialized skill and computational capabilities.
Another challenge is that multimodal AI in data analysis could potentially create privacy issues, given that it requires analyzing sensitive customer information. Companies need to guarantee compliance with data protection laws and ethical standards in using multimodal AI in data analysis.
Emerging trends and innovations in multimodal AI data analysis
The multimodal AI field is progressing very quickly, and some new trends and breakthroughs await it.
One of them is combining multimodal AI with other advancing technologies like virtual reality and augmented reality to form immersive data analysis experiences.
Progress in natural language processing and computer vision methodologies will also further refine the efficiency and accuracy of multimodal AI for data analysis.
Conclusion
In summary, the combination of visual and speech analytics using multimodal AI can unleash the full power of data analysis. By merging these two modalities, companies can derive complete insights, reveal concealed patterns, and make sound decisions.
Nevertheless, the application of multimodal AI in data analysis needs to be planned carefully, the right tools and technologies must be used, and best practices must be followed.
With each advancing wave in multimodal AI, enterprises will increasingly anticipate stronger and smarter methods for expanding their data analytical strength.